The Hugging Face đ€ Data Measurements Tool
We created the Hugging Face đ€ Data Measurements Tool, a no-code interface that helps empower members of the AI community to build, measure, and compare datasets.Â

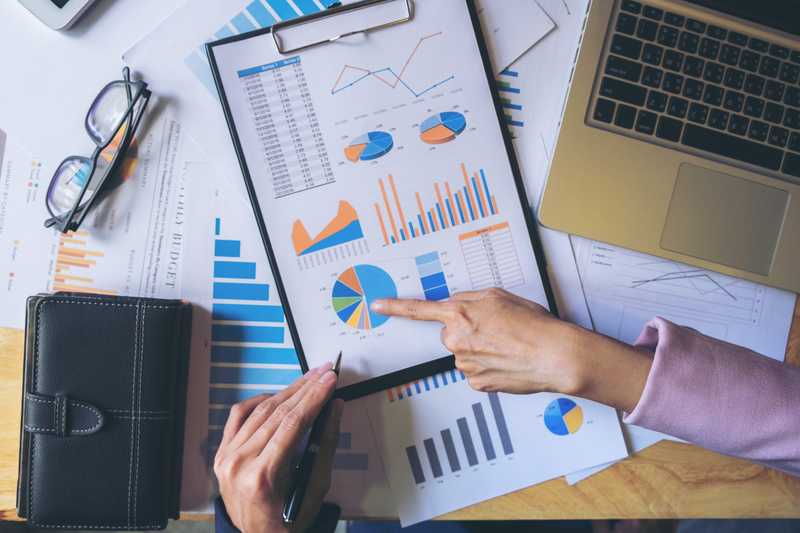
TL;DR: We created the Hugging Face đ€ Data Measurements Tool, a no-code interface that helps empower members of the AI community to build, measure, and compare datasets.Â
Exploring datasets and getting a good sense of what they contain is a key part of responsible AI practice. Gathering information about dataset labels and high-level statistics as well as honing on specific items or topics within the dataset are complementary approaches, both equally important to analyzing datasets and taking actionable steps to improve them.Â
Despite the importance of this practice, mindful dataset curation and analysis is often overlooked in AI development, with the emphasis being placed instead on improving AI models and approaches (Sambastivan et al., 2021). However, a recent wave of research has brought forward a fundamental paradigm shift in how the field approaches datasets, including better requirements for dataset development (Hutchinson et al., 2020, Paullada et al., 2020), curation (Yang et al., 2019, Prabhu and Birhane, 2020), and documentation (Gebru et al., 2018, Mitchell et al., 2019).Â
This work has inspired us to create the Hugging Face đ€ Data Measurements Tool, a no-code online tool that can be used to build, measure, and compare datasets. In the paragraphs below, we present insights and discoveries that the tool has enabled us to make with regards to popular AI datasets, and use cases in which it can be used to help members of the AI community learn more about their favorite datasets and to give them insights about their structure and contents. After reading, we encourage you to try the tool for yourself!
We started by applying the Data Measurements Tool to SQuAD, a prominent question answering dataset. Letâs look at some of the descriptive characteristics we discovered, like the number of duplicates it contains, and its shortest and longest entries.Â
Duplicate entries
We can see that SQuAD has 6 instances of questions that are literally âI couldnât come up with another questionâ, undoubtedly submitted by crowdsourcing contributors who ran out of ideas:Â
Short entries
It also has over a dozen questions that are one or two letters long, and yet remain part of the dataset.
Interestingly, the second version of the SQuAD dataset no longer contains these duplicates, and many of the extremely short questions were removed:
Itâs really useful to be able to discover things like this before using a dataset, even one as popular as SQuAD, because they can impact the quality of models that are trained on it. The noisy data points can then be filtered out or improved on via iterative model development, ultimately resulting in higher-quality datasets and better-performing models.
Dataset Labels
Suppose that you want to train a hate speech detection model, and for that you want to use two common datasets on this topic, Hate Speech 18, and Hate Speech Offensive. But can you use them both in the same model?
Actually, itâs not that easy, since the Data Measurements Tool shows us that they have different labeling schemes! While one makes the distinction between âoffensive languageâ and âhate speechâ, the other doesnât. So training a model on both datasets would be complicated!
Dataset Topics
Letâs look at another use case â you have a dataset of comments in a forum and you want to identify people talking about similar topics to see how diverse it is. How can you do that?Â
Understanding the diversity of a dataset can be challenging, but grouping the entries based on a measure of similarity can help you better understand its distribution. It turns out that by using embeddings from a Sentence-Transformer model, itâs possible to divide data points into hierarchical clusters, which are grouped around a given topic or category. This can help you find common themes and patterns in your dataset which can help you determine the datasetâs structure.Â
For instance, in a dataset from a forum like Hate Speech 18, itâs easy to find the different ways in which people greet each other:Â
In a general knowledge dataset like WikiText, you can find groups of articles around similar topics, such as these ones about astronomy:
The Data Management Tool also allows you to enter the text of your choice to see what clusters and data points are most similar to it. In the example below, we search the IMDB dataset, which consists of movie reviews, for entries that are related to âcomedyâ and we can see that several clusters of entries refer to movies that are funny and hilarious â we can then explore these clusters specifically to look at the specific movies that are deemed to be funniest by reviewers.Â
Dataset BiasesÂ
Finally, how can you see what kinds of topics, biases, and associations are in your dataset? By using the Data Measurement Toolâs normalized pointwise mutual information (nPMI) measure, you can identify stereotypes and prejudices for and against specific identity groups and populations by looking at the top words correlated with given terms such as âmanâ and âwomanâ, or âstraightâ and âgay.
In the example above from a a toxicity detection dataset, you can see that there is a skew in the dataset, since the words correlated with âwomanâ are âhomeâ, âgood (looking)â and âbeatenâ, whereas those for âmanâ are words like âyoungâ, âblacksâ and âcrimeâ, meaning that these two groups are represented very differently in this dataset.
Next Steps
We have currently developed the alpha version of the tool, showing how it can be used on popular datasets like C4, SQuAD and IMDB, to both analyze single datasets and compare two datasets side-by-side.
In the coming weeks, we will be extending the tool to cover more languages and datasets, to allow users to upload their own datasets, and to add other features and functionalities to the tool.
Our ultimate goal is to allow all those interested in and concerned by dataset quality to be able to explore and compare datasets without needing in-depth coding and technical skills. Given the democratization of AI and its growing popularity, we hope that a more mindful, data-aware approach will be adopted by our community.