Decreasing Annotation Burden of Pairwise Comparisons with Human-in-the-Loop Sorting: Application in Medical Image Artifact Rating
Ikbeom Jang, Garrison Danley, Ken Chang, Jayashree Kalpathy-Cramer Ranking by pairwise comparisons has shown improved reliability over ordinal classification. However, as the annotations of pairwise comparisons scale quadratically, this becomes less practical when the dataset is large. We propose a method for reducing the number of pairwise comparisons required to rank by a quantitative metric,… Continue reading Decreasing Annotation Burden of Pairwise Comparisons with Human-in-the-Loop Sorting: Application in Medical Image Artifact Rating
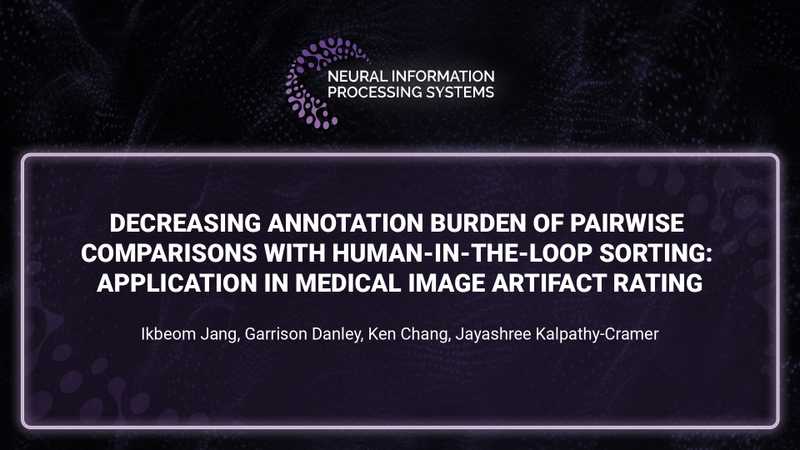
Ikbeom Jang, Garrison Danley, Ken Chang, Jayashree Kalpathy-Cramer
Ranking by pairwise comparisons has shown improved reliability over ordinal classification. However, as the annotations of pairwise comparisons scale quadratically, this becomes less practical when the dataset is large. We propose a method for reducing the number of pairwise comparisons required to rank by a quantitative metric, demonstrating the effectiveness of the approach in ranking medical images by image quality in this proof of concept study. Using the medical image annotation software that we developed, we actively subsample pairwise comparisons using a sorting algorithm with a human rater in the loop. We find that this method substantially reduces the number of comparisons required for a full ordinal ranking without compromising inter-rater reliability when compared to pairwise comparisons without sorting.